Geena V. Ildefonso, Ph.D.
As a first-generation, female scientist to graduate college, it was beyond my wildest dreams to attain a PhD. My scientific journey has motivated my passion and and excitement about developing algorithms and models for understanding complex biological systems in collaborative scientific environments.
I received a BS in Mathematics from the University of Central Florida in 2015, and Ph.D. in Systems Biology from Vanderbilt University in 2022. As a computational Ph.D. student in Dr. Carlos Lopez and Dr. Vito Quaranta's labs, I worked at the intersection of bottom-up model construction and analysis, and top-down development of computational techniques to understand and analyze cellular heterogeneity using data-driven NGS data analysis and machine learning methods.
As a graduate student, I co-developed a "Cancer Systems Biology" graduate course to foster scientific discovery in systems biology from experimental design to computational analysis with emphasis on computational modeling and machine learning. I have always had an interest in developing cross-disciplinary collaborations to bridge the scientific barrier between experimental and computational scientists.
As a postdoctoral scholar in Stacey Finley's lab at the University of Southern California, I developed Python-based workflows to computationally model the complex multi-cellular ecosystem of the tumor microenvironment in colorectal cancer using multi-omics data.
Over the course of my scientific career, I've contributed to 7 journal articles, and given 13 oral presentations at professional conferences. I recently completed an advanced whole transcriptome analysis training course at the European Molecular Biology Laboratory (EMBL) to further my scientific training. I was also chosen as a 2023 Rising Star Scholar at Sanford Burnham Prebys for exceptional postdoctoral candidates from across the country to foster greater diversity in the biomedical research and next generation of scientific leaders.
Computational Biologist | DEI Advocate | Coffee Connoisseur
Background
"If at first you don't succeed; call it version 1.0!"
Scientific Portfolio
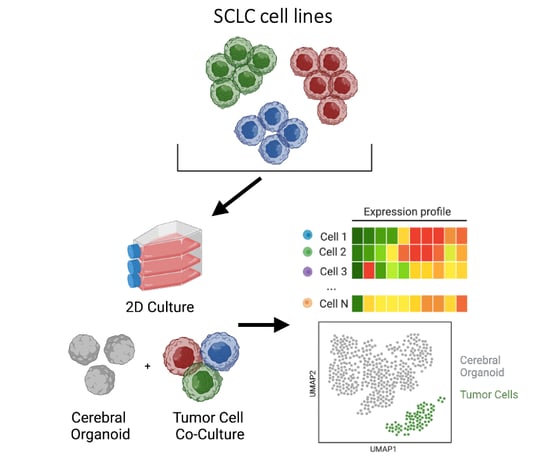
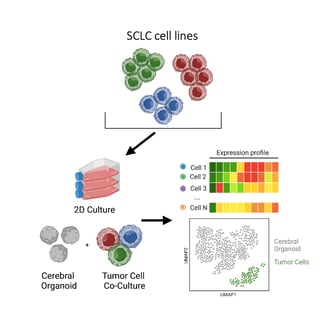
Single-cell analyses reveal the role of the brain microenvironment on small cell lung cancer subtype transitions using 3D organoids
SCLC brain metastasis remains a major cause of morbidity and mortality in SCLC patients, yet the underlying mechanisms remain poorly understood with no therapeutic strategies to prevent metastatic spread or specifically treat SCLC in the brain. Here, we utilized novel methods including PIP-seq and 3D cerebral organoids, to mimic SCLC in the brain microenvironment to assess potential phenotypic and transcriptional changes in the metastatic setting. I implemented and developed custom scripts and utilized existing tools to develop a bioinformatics pipeline for processing and analyzing multiple single cell RNA sequencing datasets from 3D organoids. We specifically identified tumor cells within cerebral organoid co-cultures, uncovered novel phenotypic subtype changes, and proposed a cellular mechanism by which SCLC subtypes transition collectively towards a YAP1+ neuronal-like state, contributing to key aspects of brain metastasis. Manuscript in preparation.
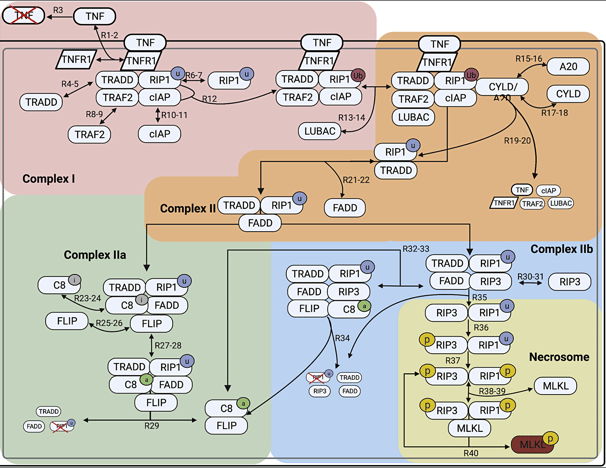
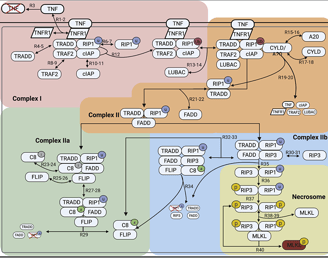
A data-driven Boolean model explains memory subsets and evolution in CD8+ T cell exhaustion
T cells play a key role in a variety of immune responses, including infection and cancer. Failure to clear antigens causes prolonged stimulation of CD8+ T cells, ultimately leading to T cell exhaustion (TCE). The functional and phenotypic changes that occur during CD8+ T cell differentiation are well characterized, but the underlying gene expression state changes are not completely understood. Here, I adapted a data-driven Boolean model of gene regulatory interactions developed from single cell RNA-sequencing data and perform a comprehensive network analysis and reveal the final gene expression states that correspond to TCE, along with the sequence of gene expression patterns that give rise to those final states. With this model we can evaluate strategies to inhibit the exhausted state. We demonstrate that a common pathway model of CD8+ T cell gene regulatory interactions can provide insights into the transcriptional changes underlying the evolution of cell states in TCE. Link to paper
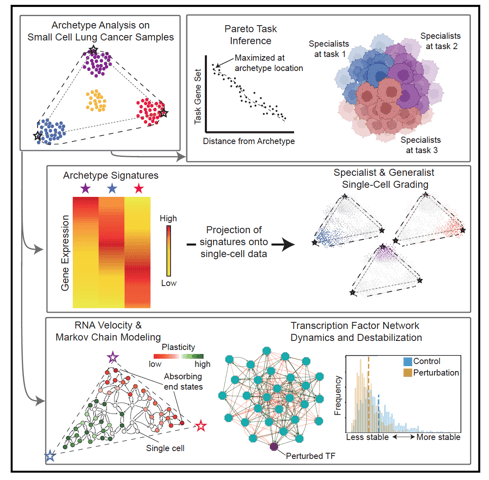
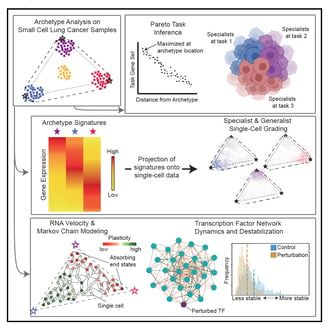
Archetype tasks link intratumoral heterogeneity to plasticity in small cell lung cancer (SCLC)
SCLC tumors are comprised of heterogenous mixtures of cell states, categorized by four key transcription factor markers, including two neuroendocrine (NE) markers: ASCL1, NEUROD1, and two non-neuroendocrine (non-NE) markers: POU2F3, and YAP1. NE to non-NE state transitions, fueled by plasticity, likely underly the adaptability to treatment and dismal survival rates. In this work, I contributed to the bulk and single cell transcriptomics data analysis to build a comprehensive understanding of the phenotypic drivers for SCLC using machine learning methods. We applied a multi-task evolutionary theory using archetype analysis (AA), proposed as an avenue to produce systems frameworks of tumor heterogeneity. I performed RNA velocity on multiple datasets, including human tumors, cell lines, and GEMM tumors, to propose a continuous view of SCLC heterogeneity based on SCLC archetypes defined by functional tasks. Link to paper
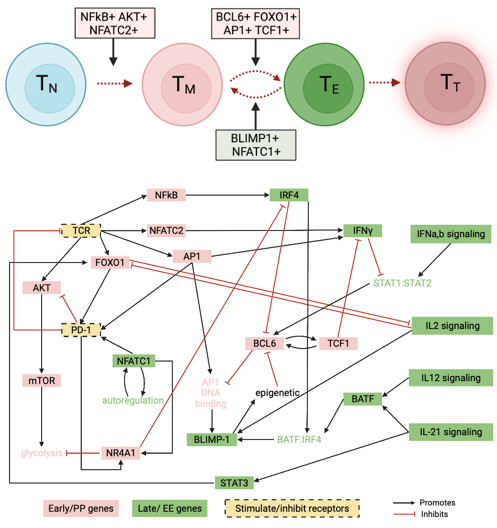
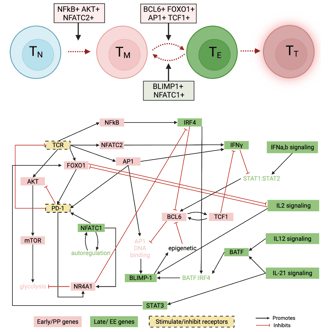
Necroptosis is an alternative form of programmed cell death, in which the cell membrane is ruptured, leading to immune response activation. In this work, I developed a non-compartmental mathematical model to simulate the dynamics of necroptosis execution upon TNF stimulation and predict modes of signal execution. I Integrated experimental proteomics and western blot data with mathematical equations to gain a deeper understanding of understanding of the basic biochemical mechanisms underlying necroptosis. We associate numerous contrasting and, in some cases, counterintuitive experimental results reported in the literature with one or more of the model-predicted modes of necroptosis execution. We demonstrated that a consensus pathway model of TNF-induced necroptosis can provide insights into unresolved controversies regarding the molecular mechanisms driving necroptosis execution in numerous cell types under different experimental conditions. Link to paper
A biochemical necroptosis model explains cell-type-specific responses to cell death cues
Featured Articles
2023 Sanford Burnham Rising Star Scholar:
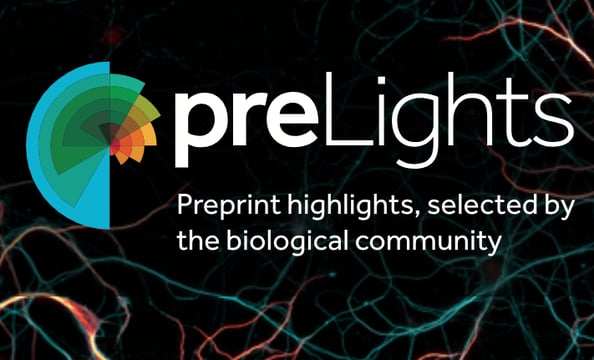
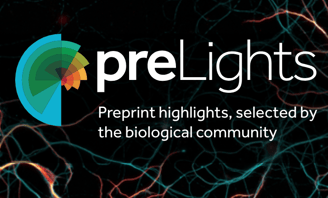
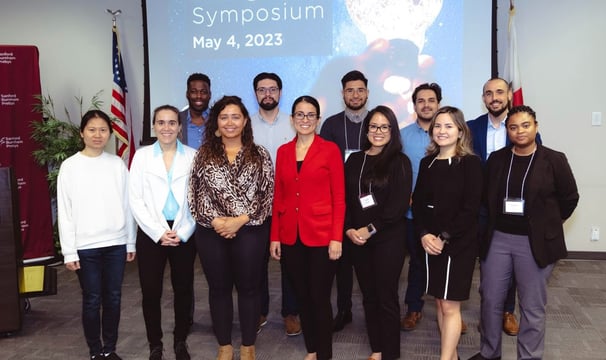
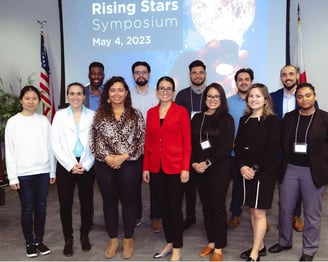
preLight preprint highlight for Boolean model:
Tool Development Contributions
Over the course of my scientific career, I had the opportunity to be a part of multiple collaborative tool development contributions.
Python Dynamic analysis of biochemical NetwOrks (PyDyNo) is an open source python library for the analysis of signal execution in network-driven biological processes.
Python Systems Biology (PySB) is a framework for building mathematical rule-based models of biochemical systems as Python programs.
Python Visualization of Processes and Reactions (PyViPR) is an ipython widget for interactively visualizing systems biology models.
Each icon has a link that will provide more information about each tool.
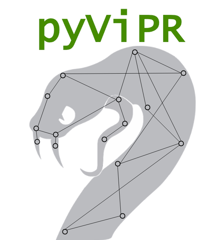
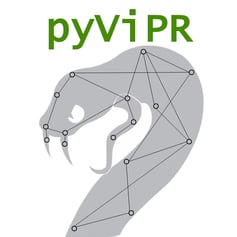
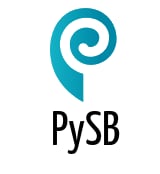
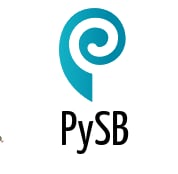
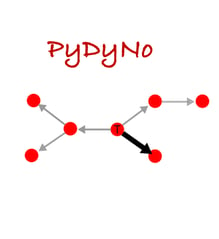
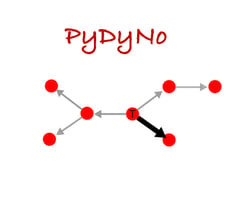
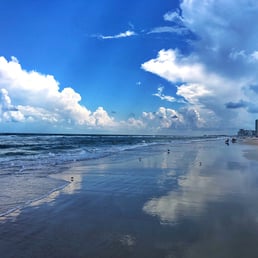
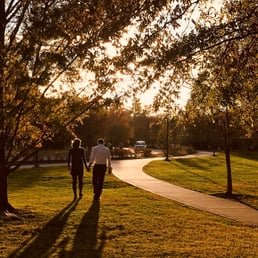
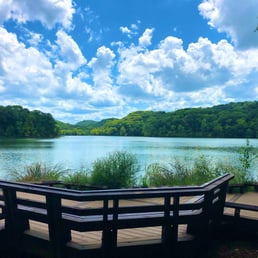
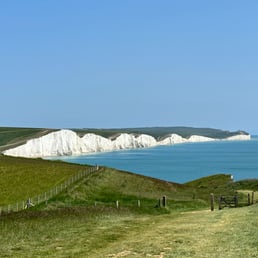
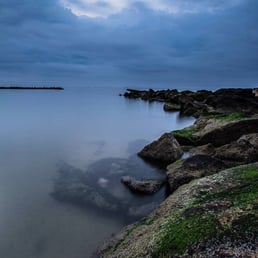
Selected Photography
Contact Me
Get in touch with Geena V. Ildefonso, Ph.D.